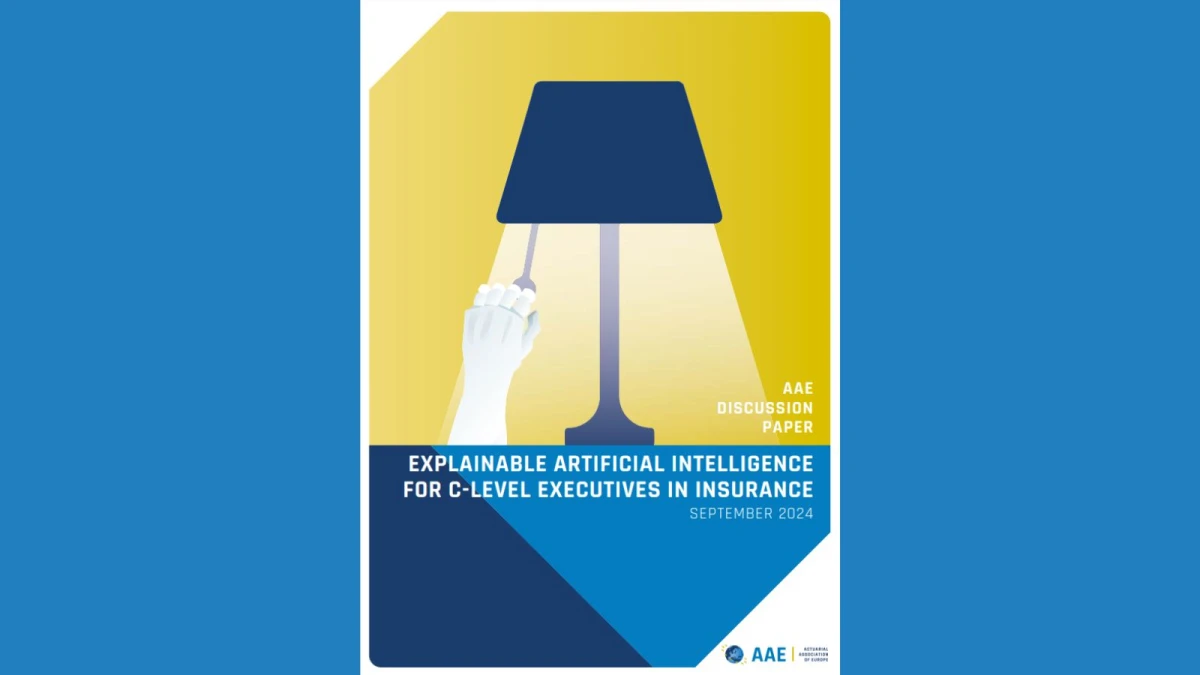
Explainable Artificial Intelligence for C-level Executives in Insurance
By demystifying AI-driven decision-making processes, XAI not only aids in adhering to the EU’s AI Act and GDPR but also elevates AI from a ‘black box’ to a more transparent, trustworthy tool. The paper provides a comprehensive toolbox of XAI methods, such as LIME, ICE, and SHAP, offering practical guidance for integrating XAI into business strategies. Executives will gain insights into leveraging XAI for strategic decision-making, enhancing model management, and demonstrating a commitment to ethical AI practices, thereby positioning their organisations as industry leaders in responsible AI.
By AAE Artificial Intelligence and Data Science Working Group
1 INTRODUCTION
Artificial Intelligence (AI) is rapidly transforming industries and people’s daily lives. ‘AI systems’ perform operations characteristic of human intellect such as data analysis, planning, language understanding, object and sound recognition, learning, problem-solving, and decision-making. Embracing AI is crucial for staying competitive and preparing for the future of the insurance industry. However, the adoption of AI, particularly in sensitive areas like pricing or claims management, is often met with caution due to concerns about ethical considerations such as transparency, and accountability. The AI Act provides clarity on legal requirements for AI to be employed in the European Union, and establishes the levels of sanctions, comprehensively covering what is legally binding. Hence, it will become ever more important to make decisions made by AI traceable, understandable, and explainable to retain intellectual oversight and control.
[....]